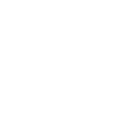
Go offline with the Player FM app!
Sakana AI - Chris Lu, Robert Tjarko Lange, Cong Lu
Manage episode 469133285 series 2803422
We speak with Sakana AI, who are building nature-inspired methods that could fundamentally transform how we develop AI systems.
The guests include Chris Lu, a researcher who recently completed his DPhil at Oxford University under Prof. Jakob Foerster's supervision, where he focused on meta-learning and multi-agent systems. Chris is the first author of the DiscoPOP paper, which demonstrates how language models can discover and design better training algorithms. Also joining is Robert Tjarko Lange, a founding member of Sakana AI who specializes in evolutionary algorithms and large language models. Robert leads research at the intersection of evolutionary computation and foundation models, and is completing his PhD at TU Berlin on evolutionary meta-learning. The discussion also features Cong Lu, currently a Research Scientist at Google DeepMind's Open-Endedness team, who previously helped develop The AI Scientist and Intelligent Go-Explore.
SPONSOR MESSAGES:
***
CentML offers competitive pricing for GenAI model deployment, with flexible options to suit a wide range of models, from small to large-scale deployments. Check out their super fast DeepSeek R1 hosting!
https://centml.ai/pricing/
Tufa AI Labs is a brand new research lab in Zurich started by Benjamin Crouzier focussed on o-series style reasoning and AGI. They are hiring a Chief Engineer and ML engineers. Events in Zurich.
Goto https://tufalabs.ai/
***
* DiscoPOP - A framework where language models discover their own optimization algorithms
* EvoLLM - Using language models as evolution strategies for optimization
The AI Scientist - A fully automated system that conducts scientific research end-to-end
* Neural Attention Memory Models (NAMMs) - Evolved memory systems that make transformers both faster and more accurate
TRANSCRIPT + REFS:
https://www.dropbox.com/scl/fi/gflcyvnujp8cl7zlv3v9d/Sakana.pdf?rlkey=woaoo82943170jd4yyi2he71c&dl=0
Robert Tjarko Lange
https://roberttlange.com/
Chris Lu
https://chrislu.page/
Cong Lu
https://www.conglu.co.uk/
Sakana
https://sakana.ai/blog/
TOC:
1. LLMs for Algorithm Generation and Optimization
[00:00:00] 1.1 LLMs generating algorithms for training other LLMs
[00:04:00] 1.2 Evolutionary black-box optim using neural network loss parameterization
[00:11:50] 1.3 DiscoPOP: Non-convex loss function for noisy data
[00:20:45] 1.4 External entropy Injection for preventing Model collapse
[00:26:25] 1.5 LLMs for black-box optimization using abstract numerical sequences
2. Model Learning and Generalization
[00:31:05] 2.1 Fine-tuning on teacher algorithm trajectories
[00:31:30] 2.2 Transformers learning gradient descent
[00:33:00] 2.3 LLM tokenization biases towards specific numbers
[00:34:50] 2.4 LLMs as evolution strategies for black box optimization
[00:38:05] 2.5 DiscoPOP: LLMs discovering novel optimization algorithms
3. AI Agents and System Architectures
[00:51:30] 3.1 ARC challenge: Induction vs. transformer approaches
[00:54:35] 3.2 LangChain / modular agent components
[00:57:50] 3.3 Debate improves LLM truthfulness
[01:00:55] 3.4 Time limits controlling AI agent systems
[01:03:00] 3.5 Gemini: Million-token context enables flatter hierarchies
[01:04:05] 3.6 Agents follow own interest gradients
[01:09:50] 3.7 Go-Explore algorithm: archive-based exploration
[01:11:05] 3.8 Foundation models for interesting state discovery
[01:13:00] 3.9 LLMs leverage prior game knowledge
4. AI for Scientific Discovery and Human Alignment
[01:17:45] 4.1 Encoding Alignment & Aesthetics via Reward Functions
[01:20:00] 4.2 AI Scientist: Automated Open-Ended Scientific Discovery
[01:24:15] 4.3 DiscoPOP: LLM for Preference Optimization Algorithms
[01:28:30] 4.4 Balancing AI Knowledge with Human Understanding
[01:33:55] 4.5 AI-Driven Conferences and Paper Review
217 episodes
Manage episode 469133285 series 2803422
We speak with Sakana AI, who are building nature-inspired methods that could fundamentally transform how we develop AI systems.
The guests include Chris Lu, a researcher who recently completed his DPhil at Oxford University under Prof. Jakob Foerster's supervision, where he focused on meta-learning and multi-agent systems. Chris is the first author of the DiscoPOP paper, which demonstrates how language models can discover and design better training algorithms. Also joining is Robert Tjarko Lange, a founding member of Sakana AI who specializes in evolutionary algorithms and large language models. Robert leads research at the intersection of evolutionary computation and foundation models, and is completing his PhD at TU Berlin on evolutionary meta-learning. The discussion also features Cong Lu, currently a Research Scientist at Google DeepMind's Open-Endedness team, who previously helped develop The AI Scientist and Intelligent Go-Explore.
SPONSOR MESSAGES:
***
CentML offers competitive pricing for GenAI model deployment, with flexible options to suit a wide range of models, from small to large-scale deployments. Check out their super fast DeepSeek R1 hosting!
https://centml.ai/pricing/
Tufa AI Labs is a brand new research lab in Zurich started by Benjamin Crouzier focussed on o-series style reasoning and AGI. They are hiring a Chief Engineer and ML engineers. Events in Zurich.
Goto https://tufalabs.ai/
***
* DiscoPOP - A framework where language models discover their own optimization algorithms
* EvoLLM - Using language models as evolution strategies for optimization
The AI Scientist - A fully automated system that conducts scientific research end-to-end
* Neural Attention Memory Models (NAMMs) - Evolved memory systems that make transformers both faster and more accurate
TRANSCRIPT + REFS:
https://www.dropbox.com/scl/fi/gflcyvnujp8cl7zlv3v9d/Sakana.pdf?rlkey=woaoo82943170jd4yyi2he71c&dl=0
Robert Tjarko Lange
https://roberttlange.com/
Chris Lu
https://chrislu.page/
Cong Lu
https://www.conglu.co.uk/
Sakana
https://sakana.ai/blog/
TOC:
1. LLMs for Algorithm Generation and Optimization
[00:00:00] 1.1 LLMs generating algorithms for training other LLMs
[00:04:00] 1.2 Evolutionary black-box optim using neural network loss parameterization
[00:11:50] 1.3 DiscoPOP: Non-convex loss function for noisy data
[00:20:45] 1.4 External entropy Injection for preventing Model collapse
[00:26:25] 1.5 LLMs for black-box optimization using abstract numerical sequences
2. Model Learning and Generalization
[00:31:05] 2.1 Fine-tuning on teacher algorithm trajectories
[00:31:30] 2.2 Transformers learning gradient descent
[00:33:00] 2.3 LLM tokenization biases towards specific numbers
[00:34:50] 2.4 LLMs as evolution strategies for black box optimization
[00:38:05] 2.5 DiscoPOP: LLMs discovering novel optimization algorithms
3. AI Agents and System Architectures
[00:51:30] 3.1 ARC challenge: Induction vs. transformer approaches
[00:54:35] 3.2 LangChain / modular agent components
[00:57:50] 3.3 Debate improves LLM truthfulness
[01:00:55] 3.4 Time limits controlling AI agent systems
[01:03:00] 3.5 Gemini: Million-token context enables flatter hierarchies
[01:04:05] 3.6 Agents follow own interest gradients
[01:09:50] 3.7 Go-Explore algorithm: archive-based exploration
[01:11:05] 3.8 Foundation models for interesting state discovery
[01:13:00] 3.9 LLMs leverage prior game knowledge
4. AI for Scientific Discovery and Human Alignment
[01:17:45] 4.1 Encoding Alignment & Aesthetics via Reward Functions
[01:20:00] 4.2 AI Scientist: Automated Open-Ended Scientific Discovery
[01:24:15] 4.3 DiscoPOP: LLM for Preference Optimization Algorithms
[01:28:30] 4.4 Balancing AI Knowledge with Human Understanding
[01:33:55] 4.5 AI-Driven Conferences and Paper Review
217 episodes
All episodes
×Welcome to Player FM!
Player FM is scanning the web for high-quality podcasts for you to enjoy right now. It's the best podcast app and works on Android, iPhone, and the web. Signup to sync subscriptions across devices.